AI Quality Control - Transforming Precision and Efficiency
Harnessing AI for Superior Quality Assurance
Discover how AI is transforming quality assurance in diverse industries. AI Quality Control brings unparalleled accuracy and efficiency, automating inspections and predicting maintenance needs. Dive into how AI is not just enhancing quality processes but also innovating and streamlining operations for the future.
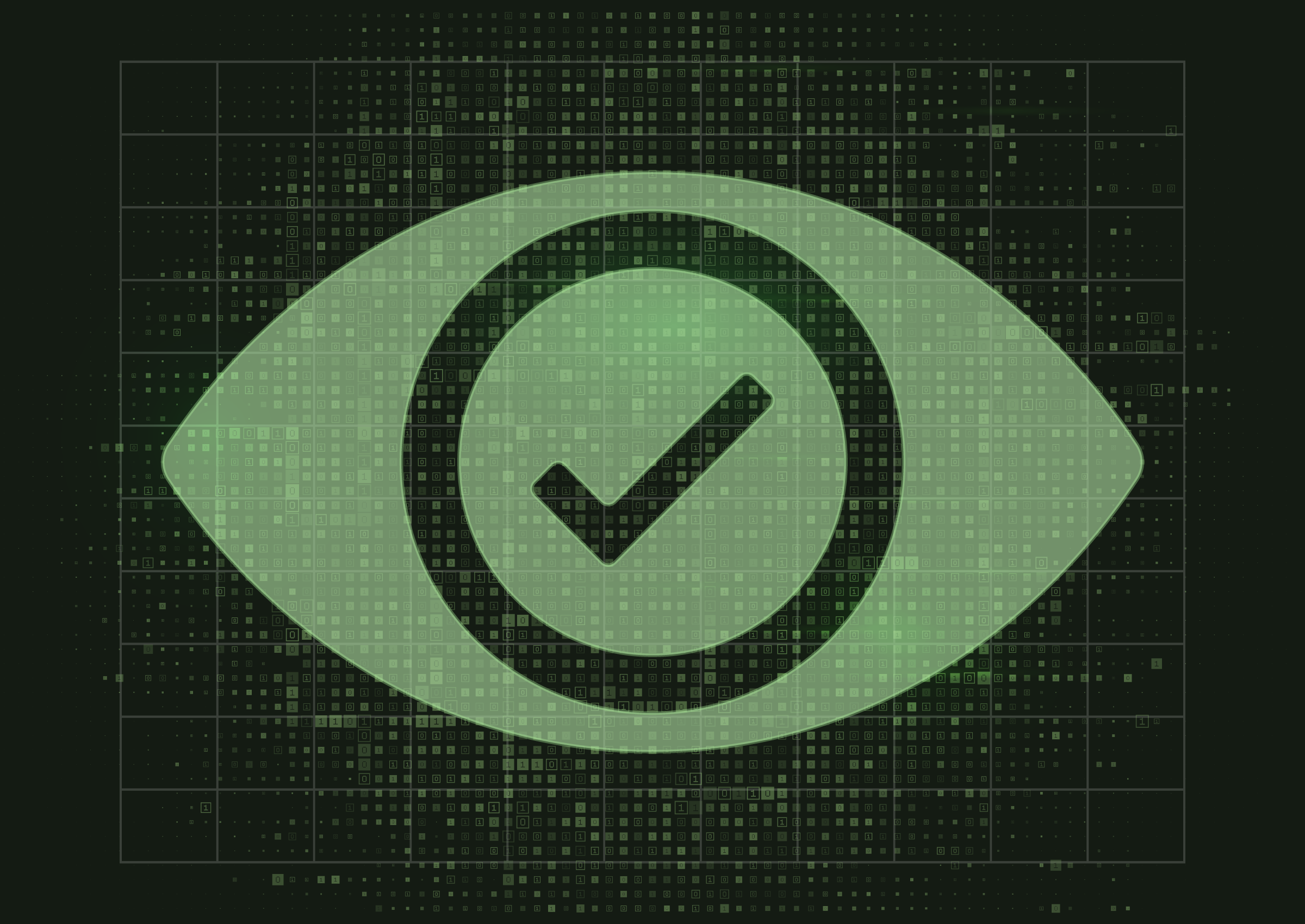
What is AI Quality Control?
AI Quality Control refers to the application of artificial intelligence in monitoring and improving the quality of products and processes. This advanced approach to quality assurance leverages AI technologies, such as machine learning, computer vision, and predictive analytics, to enhance the precision, efficiency, and reliability of quality control processes.
Key Elements of AI Quality Control
In essence, AI Quality Control represents a paradigm shift in quality assurance, moving from reactive problem-solving to proactive quality management. It’s about harnessing the power of AI to set new standards in quality and operational excellence across various industries.
Automated Inspection
AI algorithms, particularly in computer vision, are used to inspect products and components at high speed with great accuracy, identifying defects that might be missed by the human eye.
Predictive Maintenance
Machine learning models analyze data from machinery and equipment to predict potential failures before they occur, ensuring uninterrupted and optimal production processes.
Data-Driven Quality Decisions
AI Quality Control systems analyze vast amounts of data to identify trends and patterns, facilitating informed decision-making that can prevent quality issues and improve overall process efficiency.
Continuous Learning
AI systems continually learn from new data and experiences, constantly improving their ability to detect and respond to quality-related challenges.
Key Technologies and Tools in AI Quality Control
AI Quality Control relies on a range of sophisticated technologies and tools designed to improve and automate quality assurance processes. Here are five key technologies and tools integral to AI Quality Control.
Machine Learning Algorithms
Machine learning algorithms are central to AI Quality Control, enabling the analysis of complex data patterns to predict quality issues and optimize processes. They adapt and improve over time, enhancing their predictive accuracy.
Computer Vision Systems
These systems use image recognition to inspect and analyze products on production lines. They can detect defects, irregularities, and deviations from standards with high precision and speed.
Predictive Analytics Software
Predictive analytics tools use historical and real-time data to forecast potential quality issues, allowing for proactive maintenance and quality control measures.
AI-Powered Inspection Robots
These robots integrate AI algorithms with robotic technology to conduct thorough and accurate quality inspections, especially in environments challenging for humans.
Data Management Platforms
Robust data management platforms are essential for collecting, storing, and processing the vast amounts of data generated in AI Quality Control processes. They ensure data integrity and accessibility for AI applications.
Challenges and Solutions in AI Quality Control
Implementing AI in quality control can be challenging, but understanding and addressing these challenges is key to leveraging its full potential. Here are three significant challenges and their solutions.
Integration with Existing Systems
Seamless Integration Strategies
Data Quality and Quantity
Robust Data Management and Augmentation
Keeping Up with Technological Advances
Continuous Learning and Adaptation
Future of AI Quality Control
The field of AI Quality Control is continuously advancing, shaping the future of quality assurance in various industries. As we look forward to what lies ahead, here are three key takeaways that highlight the direction and potential impact of AI in quality control.
Greater Automation and Precision
Future advancements in AI Quality Control will likely focus on achieving even higher levels of automation and precision. Enhanced machine learning algorithms and more sophisticated computer vision systems will allow for near-perfect accuracy in defect detection and process optimization, reducing human error and increasing efficiency.
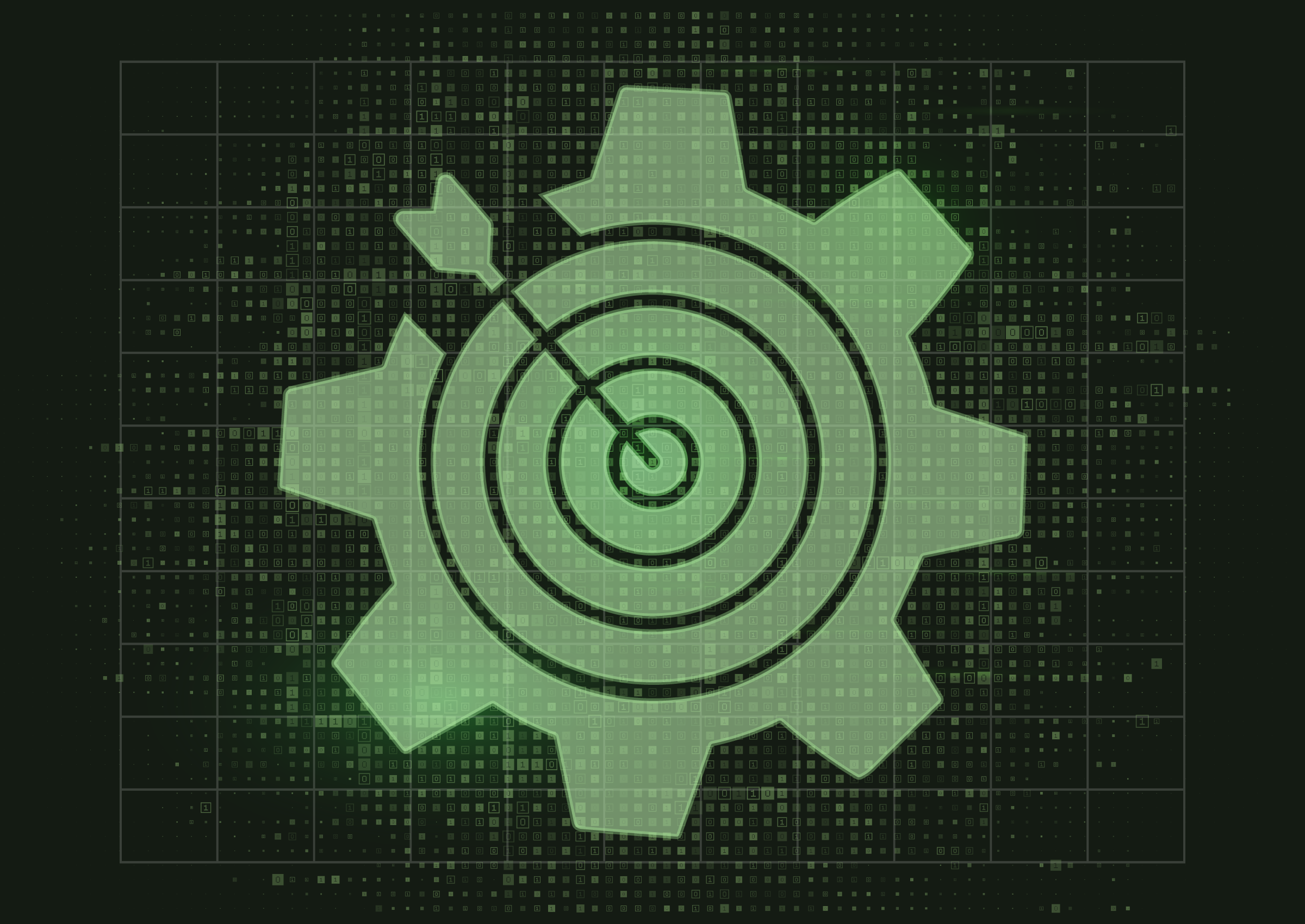
Integration of IoT and Real-time Analytics
The integration of the Internet of Things (IoT) with AI Quality Control systems will enable real-time monitoring and analytics of production processes. This will facilitate immediate adjustments and improvements, leading to smarter, more responsive quality assurance practices.
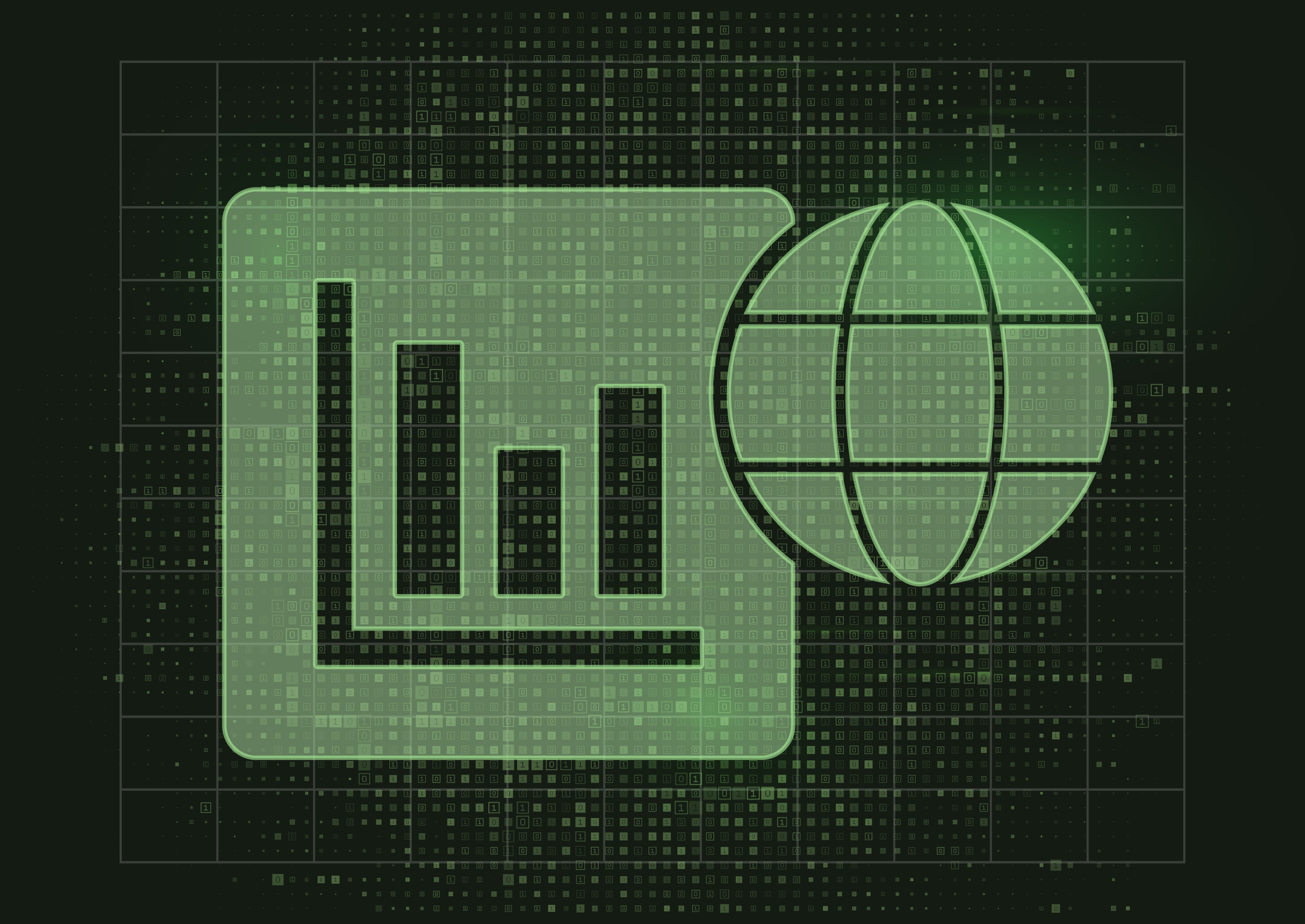
Ethical AI and Transparency
As AI becomes more prevalent in quality control, there will be a growing emphasis on ethical AI practices and algorithmic transparency. This focus will ensure that AI systems in quality control are fair, unbiased, and accountable, enhancing trust and reliability in AI-driven processes.
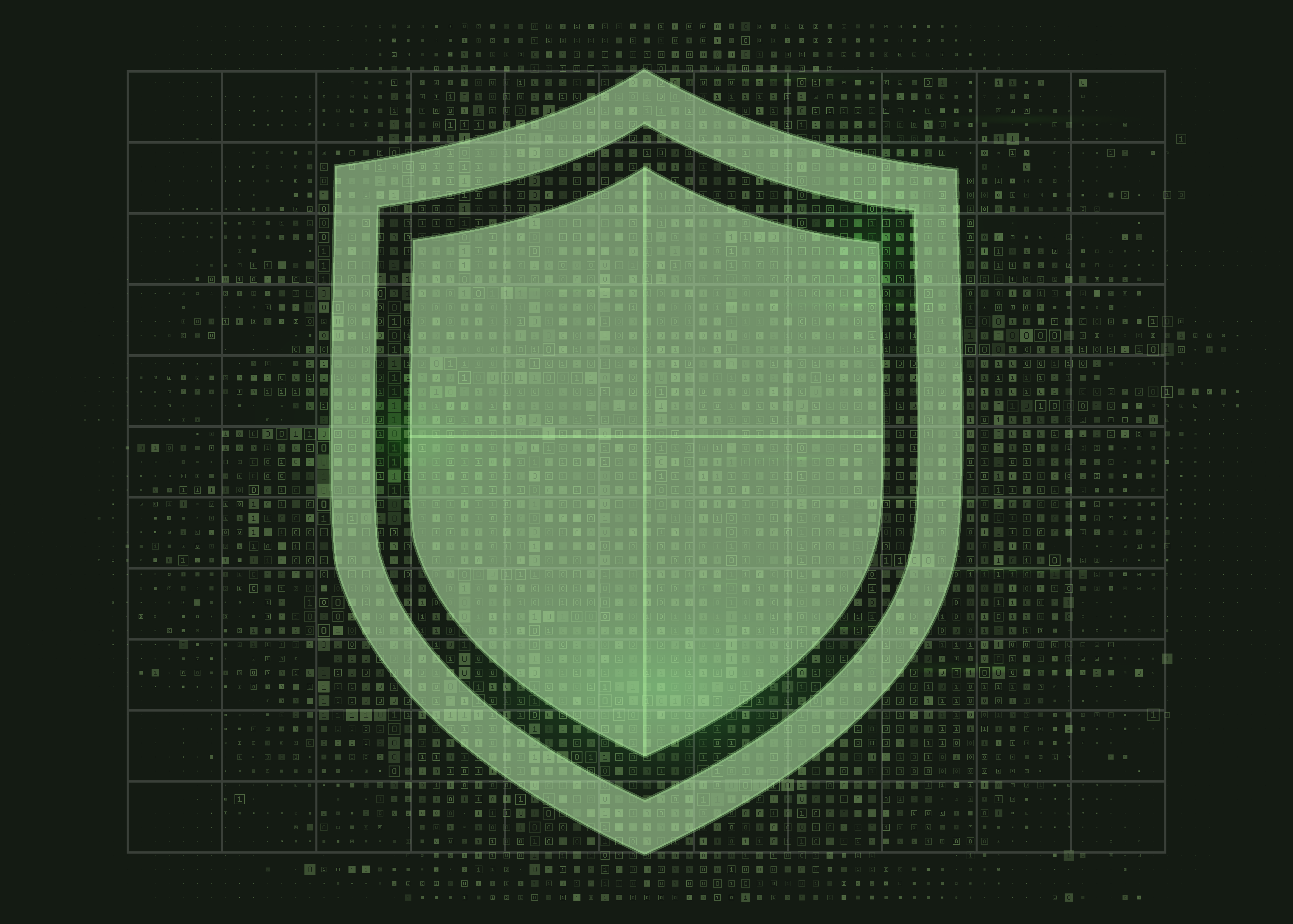
Learn more
Intrigued by the potential of AI in revolutionizing quality control processes? Keen to explore how these advanced technologies can transform your quality assurance strategies?